In a sunlit greenhouse outside Toronto, rows of strawberry plants stand in neat lines atop raised benches. They aren’t the typical berry bushes of California fields or pick-your-own farms. These strawberries are part of a bold experiment in agriculture – one that merges the age-old art of plant breeding with cutting-edge artificial intelligence. The goal is as ambitious as it sounds: to breed a sweeter, hardier, and more sustainable strawberry, and to do it faster than ever before. It’s a vision that has brought together an unlikely trio of partners and sparked fresh conversation about the future of our food.
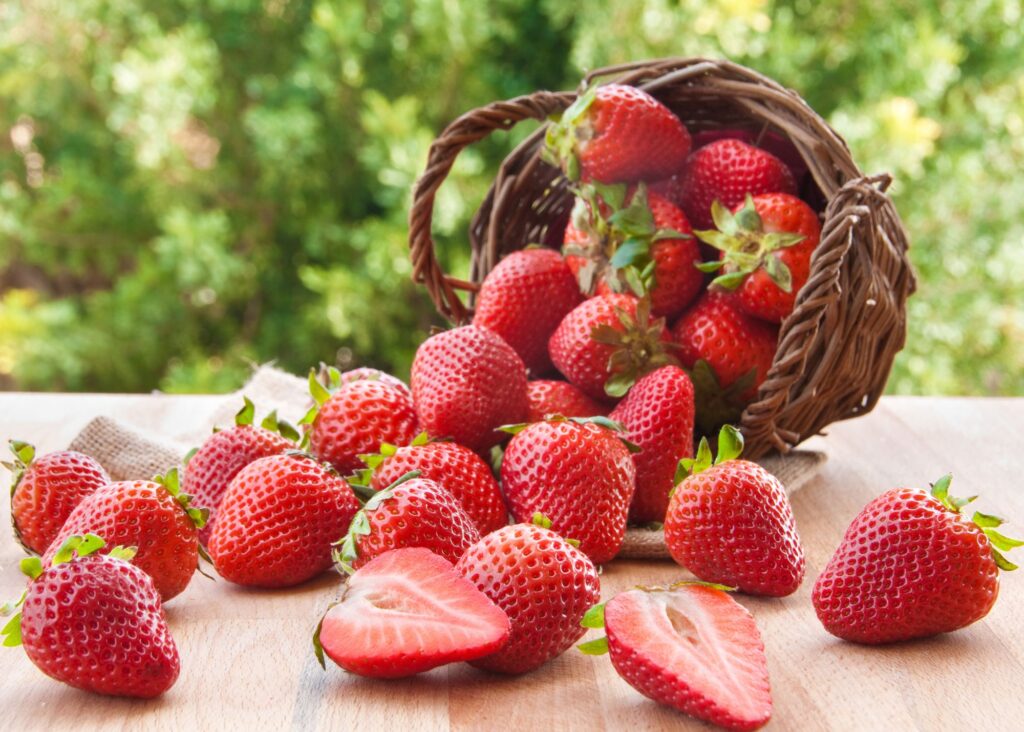
Table of Contents
ToggleAn Unlikely Alliance for a Better Berry
Earlier this year, a partnership was announced that made both farmers and tech investors perk up. Heritable Agriculture, a California-based startup born out of Alphabet’s famed X “moonshot” lab, has teamed up with Italy’s Consorzio Italiano Vivaisti (CIV) – a venerable strawberry breeding institute – and Paul J. Mastronardi, a leading figure in North American greenhouse farming. On the surface, each brings something essential to the table. CIV contributes over 40 years of strawberry germplasm (the living genetic library of berry plants) and deep breeding know-how. Heritable offers AI-driven breeding models and machine learning expertise. And Paul Mastronardi, whose family name is synonymous with greenhouse-grown produce, provides the commercial scale, production facilities, and distribution network to get new strawberry varieties from the lab to supermarket shelves.
This “trifecta of expertise” is designed to forge a synergy that surpasses what any single entity could achieve alone. In traditional plant breeding, a company might have to spend years making crosses and testing seedlings, or a grower might try adapting field-bred plants to indoor systems with middling success. By uniting forces, the partners plan to shortcut those limitations. “By uniting germplasm, AI expertise, and advanced growing capabilities, this collaboration forges a synergy that surpasses what any single entity could accomplish alone,” the group declared in its announcement. It’s a collaborative approach that reflects a broader truth in agriculture: the challenges of today’s food system – from climate stress to changing consumer tastes – are too complex for any lone innovator.
What exactly will this alliance do? The immediate focus is on developing advanced strawberry varieties tailored for controlled environments like high-tech greenhouses and “tabletop” farming systems (where berries grow in elevated trays or troughs). These are settings far different from an open field. Indoors, growers can manipulate light, temperature, and nutrients, but they also face unique hurdles: pollinating without bees, preventing humidity-related diseases, and justifying the high cost of indoor infrastructure with premium quality fruit. Current strawberry breeds weren’t designed for these conditions. “Traditional breeding programmes took several years and millions of dollars to bring new cultivars to market,” the partners noted, and even then, most programs targeted field production and a narrow set of traits. The result? Berries that may have high yield on a farm, but inadequate control over essential characteristics like taste, disease resistance, and productivity under greenhouse conditions. In other words, the perfect strawberry for a sunny day in a California field might flop in a Canadian greenhouse in December.
Paul Mastronardi has seen these challenges firsthand in the push to grow berries indoors. Mastronardi’s family business helped popularize greenhouse tomatoes and peppers in North America, and he believes strawberries are next. But success will require new genetics. “Greenhouse and tabletop environments vary widely, requiring tailored genetics and distinct growing protocols to optimize performance and meet market demands,” Mastronardi explains. He notes that simply importing methods from the field won’t cut it under glass and steel. Berries need to be bred for the quirks of indoor agriculture – from how their roots feed in substrate, to how their flowers set fruit under LED lights. The partnership has already engaged with major retailers in North America to eventually bring these AI-bred strawberries to consumers, aiming for year-round supplies of premium-quality fruit that will “see farms thrive for generations”. In Mastronardi’s words, this could be “a paradigm shift” for the greenhouse produce sector.
From Cross-Pollination to Computation: Breeding Meets AI
To appreciate how novel this approach is, it helps to understand how strawberry breeding traditionally works. Plant breeding is often described as equal parts science, art, and patience. A breeder selects parent plants with desirable traits – say, a variety with great flavor and another with strong disease resistance – and cross-pollinates them. The breeder then sows hundreds or thousands of seeds from that cross and waits for the seedlings to grow and bear fruit. Most will be average; a few might combine the parents’ best traits. It can take 5 to 7 years (sometimes longer) to evaluate, select, and propagate a new strawberry variety this way, and many more years to scale up for commercial release. During that time, tastes may change, diseases may evolve, or a competitor might release something better. It’s a costly, labor-intensive gamble, and the odds of breeding that blockbuster berry are not unlike finding a needle in a haystack.
Traditional breeding also had to focus on one or two primary traits at a time – historically yield, size, or perhaps firmness for shipping – simply because measuring and improving everything at once was impractical. Flavor often took a backseat; disease resistance might be an afterthought. As a result, many modern fruits and vegetables have been jokingly described as “bred for truck travel” rather than taste. Strawberries are a case in point: consumers today sometimes complain that supermarket berries are big and hardy but often lack the sweetness and aroma of garden-grown varieties. Breeders certainly wanted better flavor and resilience, but when each breeding cycle takes years, there’s a limit to how much you can juggle at once.
This is where Heritable’s high-tech approach aims to upend the paradigm. Instead of relying on intuition and slow feedback from field trials, Heritable uses artificial intelligence to predict which plant crosses will yield the best offspring – before those offspring are ever grown. The company’s platform crunches vast datasets (from DNA sequences and trait measurements to environmental factors) to simulate breeding outcomes on a computer. In essence, it’s creating a “virtual breeding” environment where thousands of potential strawberry seedlings can be tested in silico overnight, rather than in soil over several seasons.
What does this look like in practice? The partnership outlined a few key capabilities of their AI-driven breeding program:
- Predicting crop performance through simulations before planting. Instead of planting seeds blindly, the AI models can forecast how a given genetic combination might perform – how sweet the berries might be, how big the yield, how resilient to a certain fungus – all based on patterns learned from prior data.
- Optimizing multiple traits simultaneously. The AI doesn’t just focus on yield or taste alone; it can juggle dozens of traits. For greenhouse strawberries, that means balancing flavor, aroma, texture, disease resistance, shelf life, and yield all at once – a multidimensional optimization that would overwhelm a human breeder. This could finally crack the puzzle of producing berries that are both delicious and high-yielding, without trading one for the other.
- Designing varieties for easier cultivation. Traits that make a farmer’s life easier, like plants that fruit earlier, or have a more compact shape suited to hydroponic trays, can be baked into the breeding goals. The AI might, for example, prioritize a plant architecture that allows better airflow in a greenhouse or one that is ideal for automated harvesting.
- Cutting the breeding cycle time. Perhaps most importantly, these simulations and computational predictions drastically reduce the breeding timeline, potentially saving years. By pinpointing promising crosses and even identifying the best individual seedlings in advance, the team can bring superior varieties to market faster than the traditional method ever could.
“Our approach empowers greenhouse and tabletop growers to break free from traditional breeding limitations,” says Dr. Brad Zamft, the CEO of Heritable Agriculture. Zamft, who holds a PhD in physics and once worked at the Bill & Melinda Gates Foundation on agricultural programs, founded Heritable with the conviction that advances in computation could be a game-changer for crop improvement. At Google’s X research lab, he and his team built algorithms that analyze plant genomes for combinations of genes that might yield big agronomic payoffs. For example, imagine breeding corn that requires much less water, or rice that stores extra carbon in its roots to help soil health. “By understanding those genomes, the crops can then be bred with climate-friendly traits for increased yields, lower water requirements, and higher carbon storage capacity in roots and soil,” Zamft explains. It’s a process of data-driven discovery: instead of waiting to see what nature gives you from a cross, you ask the computer to suggest the genetic recipe for the traits you need.
The implications of such technology go well beyond strawberries. Heritable’s predictive models are designed to be species-agnostic, meaning the underlying AI “brain” can in theory work on any crop, given the right data. In fact, the company has already trialed its platform on tomatoes, spinach, peppers and more, demonstrating that the approach generalizes across different plants and environments. This was a deliberate move: rather than hand-crafting a solution for one crop, Heritable built a flexible system that learns from patterns in plant biology as a whole. Much like how a language translation AI can be taught new languages, a breeding AI can be fed data on a new species and start making predictions, as long as it’s trained on enough examples. That adaptability is crucial if the goal is to revolutionize breeding across the food system.
To get those “enough examples,” Heritable and CIV are combining their knowledge repositories. CIV brings decades of observations from its breeding trials – which varieties thrived in different regions of Europe, which succumbed to pests, which produced the sweetest fruits – and, importantly, a rich collection of strawberry germplasm (essentially the stockpile of diverse strawberry genetics they’ve saved over years). The AI can leverage this trove of biological big data to discern genetic patterns. According to the company, it uses “multi-omic” data in its models. In biotech jargon, omics refers to layers of biological data: genomics (DNA sequence info), transcriptomics (gene expression data), maybe metabolomics (chemical compounds in the plant), and enviromics (environmental data). By integrating these layers, an AI model might, for example, notice that a certain gene variant coupled with a specific climate condition leads to higher vitamin C content, or that a combination of five genes gives resistance to a new strain of fungus when grown hydroponically. This kind of insight could take scientists a lifetime to piece together manually, but machine learning algorithms are adept at sniffing out complex correlations.
For the grower and the consumer, the result of this hyper-complex analysis boils down to something simple: better plants, sooner. The partnership expects their first AI-guided strawberry crosses to yield tangible results within a few years, not decades. Once promising seedlings are identified (virtually and then in real greenhouses), those plants can be cloned and tested in pilot production at Mastronardi’s facilities. Only the most outstanding performers – say a strawberry that fruits abundantly in mid-winter with excellent flavor – would move forward into commercial propagation. And because the AI can run millions of simulations to explore the breeding “design space,” there’s a higher chance of finding those rare winning combinations that traditional breeders might miss. In effect, the breeders aren’t flying blind anymore; they have a data-informed map pointing them toward the treasure.
It’s worth noting that this approach builds on a foundation laid by earlier advances in breeding. For years, plant scientists have used techniques like marker-assisted selection and genomic selection – early forms of data-driven breeding that use genetic markers to predict trait outcomes. AI takes this to the next level by handling far more variables and using modern computing power to find patterns. As one review by leading crop scientists noted, AI has already begun to “revolutionize” aspects of crop breeding such as trait prediction and gene discovery, but the real leap will come from integrating these components into a seamless pipeline. The Heritable-CIV initiative is an attempt to build exactly that kind of integrated, AI-enabled breeding pipeline. It’s essentially Breeding 4.0: combining genomics, big data, and machine learning to turbocharge what used to be an arduous, slow art. In theory, this could expedite “genetic gain” – the term breeders use for the amount of improvement achieved per breeding cycle or year. Faster genetic gain means faster progress in crop yield, nutrition, and resilience.
Rethinking Supply Chains: Year-Round Strawberries and Resilient Crops
If AI-bred strawberries fulfill their promise, the ripple effects could extend from farms to supply chains and even to global food security. Consider the humble strawberry in winter. In much of the U.S. and Canada, fresh strawberries in December traditionally come from far away – often shipped from warmer climates like California, Mexico, or Florida. That long supply chain adds cost, transportation emissions, and time (which can mean less freshness). It also concentrates production in a few regions, so a drought or flood in one area can cause price spikes nationwide. Now picture a different model: clusters of high-tech greenhouses outside every major city, producing locally-grown strawberries even during a blizzard. For consumers, it means ripe, fragrant berries year-round; for retailers, a steady, predictable supply with potentially less volatility; for the environment, fewer diesel trucks hauling fruit across continents.
This is one of the visions that enthusiasts of indoor farming have been touting. But it hinges on having the right crop varieties to make it economically viable – something this new breeding program directly addresses. “We expect to bring novel varieties to the market that will deliver a premium quality experience for the consumer and retailer, while ensuring crop economics that will see farms thrive for generations,” Paul Mastronardi said of the partnership’s goals. The crop economics part is key: indoor farming is expensive, so yields must be high and consistent, and the product quality must command a good price. A strawberry that wows consumers with aroma and taste can fetch a premium; one that also yields reliably in greenhouse conditions helps the farmer’s bottom line. By optimizing for both, the AI-bred varieties aim to square a difficult circle – delivering what the market wants and what the grower needs to profit.
The supply chain benefits could be significant. With climate change introducing more uncertainty (California’s berry fields, for example, have suffered severe swings from droughts to unseasonal floods in recent years), diversification of production through greenhouses creates a buffer. It’s a form of climate resilience: if a freak storm ruins a field harvest, indoor growers can pick up some slack. Moreover, greenhouse operations can be located closer to population centers (since they’re not tied to specific soil or weather conditions), shrinking the distance from farm to table. That reduces fuel use and can preserve more of the fruit’s shelf life and nutrients by the time it reaches stores. According to industry reports, interest in greenhouse-grown berries is soaring. In Europe and Asia, growers have led the way – the share of strawberries grown under cover has been steadily climbing. Between 2006 and the late 2010s, the percentage of strawberries grown in open fields in some countries (like the Netherlands) dropped from 85% to around 76%, as more production moved to greenhouses and other protected environments. North America is catching up: companies like Driscoll’s, the largest berry distributor, have even partnered with indoor farming startups (such as Plenty) to build vertical farms for strawberries in the U.S.. All of this signals that how and where we grow berries is on the cusp of major change.
But today’s indoor strawberry ventures have often been using off-the-shelf plant varieties, originally bred for fields. The feedback from growers has been clear – better genetics are needed to unlock the full potential of indoor systems. Different day-length responses, different pest resistances, different growth habits can make the difference between a bumper crop and a disappointing yield under glass. For instance, U.S. greenhouse growers found that European short-day strawberry varieties behaved very differently than the everbearing day-neutral types U.S. field farmers use. There was a steep learning curve and a lack of data on what works indoors. This new program directly tackles that gap, essentially developing the first generation of strawberries bred specifically for high-tech North American greenhouses.
One immediate supply chain consideration is the propagation of these new varieties. Unlike row crops such as corn or lettuce that are grown from seed, commercial strawberries are often propagated from cuttings (runners) or tissue culture plantlets. That means once a winning genotype is identified, it can be cloned and scaled relatively quickly – no need to wait for seeds. However, it also means the supply chain has to provide nurseries with disease-free starter plants to growers. As one Canadian greenhouse farmer noted, “consistent, quality transplant material is the key to high-quality greenhouse strawberries”. The industry will need to gear up to supply those transplants of the AI-bred varieties. It’s a solvable problem (nurseries already do this for existing varieties), but it underscores that innovation isn’t just about the seed or plant – it’s about the whole chain from lab to consumer. The partnership’s advantage is that it has players covering that chain: CIV on breeding, Heritable on tech, and Mastronardi on production and distribution. If all goes well, the handoff from one to the next should be relatively smooth, accelerating how fast consumers might actually taste these futuristic strawberries.
Looking at the bigger picture, strawberries are a high-value specialty crop, but the same model could be expanded to staple foods and other corners of global agriculture. In many ways, the Heritable-CIV initiative is a case study for what AI-driven breeding can do. If it works for strawberries, why not wheat, rice, or maize? Those staple grains are the backbone of global food security, and the need for improvement there is dire. The United Nations projects that by 2050, the world will need to produce roughly 50–70% more food to feed nearly 10 billion people, given current consumption trends . Yet yield gains in major crops have been plateauing. In the last few decades, annual yield increases for corn, soy, and wheat have hovered around just 1% – a rate that experts warn is not fast enough to meet future demand. “Progress in seed has stagnated,” as Inari, another agtech startup, pointed out, noting that 1% annual gains are “simply not enough” when climate extremes and population growth are taken into account. Inari’s answer is to pair AI with gene editing to boost yields by 10–20% in those big crops. Heritable’s approach with AI-driven crossing is philosophically similar – use technology to dramatically accelerate the pace of improvement.
Imagine applying AI breeding to develop drought-tolerant wheat that can thrive as rainfall patterns shift, or heat-resilient rice that can withstand hotter summers. These are not sci-fi scenarios; they are actively being pursued. In fact, large agricultural companies and research institutions worldwide are investing in data-driven breeding. The International Maize and Wheat Improvement Center (CIMMYT) and other global institutes are exploring AI tools to help resource-poor farmers get climate-resilient crop varieties faster. Companies like Bayer and Corteva (owners of legacy seed brands like Dekalb and Pioneer) have integrated advanced analytics and genomic prediction into their breeding pipelines for years – although they might use the term “predictive breeding” or “genomic selection” rather than AI. The difference now is the explosion of computational power and machine learning techniques that can handle far more complex datasets. Modern AI can analyze not just a plant’s genome, but also satellite imagery of field conditions, real-time weather data, soil microbiome profiles, and more, all together. This could help breeders identify, for example, the combination of genes that helps a crop use nitrogen more efficiently in a specific soil type, or the genomic markers that predict a plant’s yield across five different climate scenarios. One academic paper outlines “four key areas where AI holds the potential to expedite genetic gain: panel selection, generation of biological big data, biological interpretation, and phenotype prediction.” In plain English, AI can help choose better parents for crosses, make better use of massive genomic/phenomic data, interpret the biology underlying traits, and predict which plants will perform best. These are exactly the tasks breeders spend careers grappling with – and exactly what AI is being tasked to supercharge.
Heritable’s team has hinted that their platform is already being eyed for other crops beyond the initial strawberry push. “Heritable Agriculture’s multi-omic, AI-driven predictive models generalize across species, environments, and traits, making the approach highly adaptable to any operation,” the company noted, adding that the technology “extends to crops such as tomatoes, spinach, and peppers” for growers looking to diversify. It’s not hard to envision partnerships similar to the strawberry trifecta forming in other sectors: maybe an AI company working with a maize research institute in Africa to breed drought-proof corn, or collaborating with a big rice producer in Asia to tackle yield and nutrition in rice simultaneously. In fact, as a proof of concept, Heritable grew thousands of trial plants in specialized growth chambers and field plots during its incubation at Google X – including trials in California, Nebraska, and Wisconsin – to test and refine their models on diverse crops and conditions. The results gave them confidence that their algorithms can handle both controlled environments like greenhouses and the messier dynamics of open fields.
Backing the Vision: Business, Investment, and the Road Ahead
It’s no coincidence that an effort like this strawberry AI-breeding program is happening now. The convergence of ag and AI has been brewing, and serious money is pouring into it. Heritable Agriculture itself is a product of Silicon Valley’s venture engine. The startup officially spun out of Alphabet’s X division (Google’s “moonshot factory”) in early 2025 with a seed funding round backed by agtech-focused investors like FTW Ventures, Mythos Ventures, SVG Ventures, and even an equity stake from Google itself. While the exact dollar amount wasn’t disclosed in reports, these backers signal strong confidence – SVG Ventures’ Thrive fund is known for agri-food tech, and Google’s involvement suggests the tech giant sees promise in Heritable’s approach. In an era when AI startups are a dime a dozen, Heritable stands out by targeting something very tangible and high-impact: crop yields and sustainability. As TechCrunch reported, the startup’s genesis came from Zamft being given a wide brief at X to “work on whatever could scale to a Google-sized business,” and the problem of optimizing plants at scale fit the bill.
The business model here appears to be deep collaboration rather than direct competition with established players. Notice that Heritable didn’t decide to become a seed company and develop its own varieties in isolation. Instead, it partnered with a traditional breeder (CIV) and a grower/marketer (Mastronardi). This mirrors a strategy seen elsewhere in agtech: new tech companies often license their innovations or co-develop with incumbents rather than trying to displace the entire value chain. Inari, for example, the Cambridge, MA “SEEDesign” company working on AI and gene editing, explicitly supplies its trait discoveries to big seed companies instead of selling to farmers itself. “These seed companies have incredibly deep relationships with their farmers, so they know what their customers need,” Inari’s CFO Lara Smith Weber explained, underscoring why partnering made sense. Inari has raised over $720 million in funding to date, showing how much capital is betting on the combination of biotech and AI to boost crops. Heritable’s approach so far seems similarly “asset-light” – leverage the strengths of others (like CIV’s germplasm, Mastronardi’s facilities) while providing the secret sauce (the AI models). This reduces the need for Heritable to invest in its own farms or seed production infrastructure, which would be huge undertakings.
Of course, Heritable likely will secure intellectual property (patents, trade secrets) around its AI breeding methods and any novel plant varieties that result. The value captured will depend on how they structure deals: perhaps a share of royalties from any hit strawberry variety, or fees for applying their platform to other breeding programs. There’s also a hint that Heritable could scale by becoming a sort of AI breeding platform-for-hire. If their models truly generalize, they could contract with different crop breeders – say helping a lettuce company develop a downy-mildew-resistant line, or assisting a forestry company in breeding more carbon-sequestering trees. In essence, they could become a new kind of agricultural technology provider, one that sells not seeds or tractors but insights – genomic predictions as a service.
The involvement of a figure like Paul Mastronardi also indicates a business calculus: Mastronardi’s company (Mastronardi Produce, known for its Sunset® brand tomatoes, peppers, etc.) has a track record of introducing and marketing new produce varieties successfully. The Campari tomato, the Zima orange grape tomato, the Angel Sweet – these are all products Mastronardi helped popularize, changing consumer expectations of how flavorful a supermarket tomato can be. Having him on board suggests that if an AI-bred strawberry with exceptional taste comes out of this, there is already a clear path to branding it and getting it into national retail chains at scale. In other words, this isn’t just a science experiment; it’s being engineered with commercial impact in mind from day one.
Heritable’s choice to stick (for now) with conventional breeding rather than gene editing or GMOs is also a savvy business decision. Gene-edited crops are promising and can achieve things traditional breeding can’t, but they face regulatory hurdles in some markets and public acceptance questions. Zamft noted that “we’re not developing gene-edited plants, and genetic modification is not on our roadmap” at this stage. Instead, they see a “huge, unmet need for identifying what to breed and then doing better breeding – crossing a mother and father plant” the old-fashioned way, just informed by ultra-modern data tools. This means any varieties they create can be grown and sold without additional regulatory approval beyond what any new cultivar needs. It speeds up time to market and avoids the debate around GMOs. That said, Zamft acknowledged CRISPR gene editing could eventually play a role – one can imagine a future where their AI finds an ideal genetic tweak that isn’t present in any known strawberry germplasm, and then a quick edit could introduce it. But that’s further down the road. The immediate focus is to prove that AI-assisted conventional breeding can deliver results.
Opportunities and Risks of an AI-Adapted Food System
Any transformative technology comes with its share of promise and peril. As AI-driven breeding moves from hype to reality, it’s important to weigh the opportunities against the risks – biological, ethical, economic, and technological.
- Biological opportunities: We could breed crops that are dramatically more productive, nutritious, and resilient. AI can explore combinations no human would think to try, potentially uncovering traits that make plants thrive in a hotter, drier climate or that pack more vitamins into each bite. The genetic gain in breeding (how much better each new generation is) could accelerate, which is desperately needed for keeping food supplies growing. We might also be able to broaden the range of crops grown in different environments – e.g. making tropical crops adapt to temperate zones or vice versa – increasing agricultural diversity. From a global food security standpoint, faster breeding could help address crises like emerging plant diseases (think of the banana fungal blight or wheat stem rust) by staying a step ahead with resistant varieties.
- Biological risks: On the flip side, there’s a concern that AI breeding could narrow genetic diversity if not managed carefully. If many breeders rely on similar algorithms and data, they might all converge on very similar “optimal” varieties, reducing the genetic variability in farms. Agriculture has learned hard lessons about the danger of monocultures – genetic uniformity can make crops sitting ducks for a new disease or pest. Maintaining a broad genetic base and wild crop relatives as reserves will be crucial, even as we hone elite varieties. Another risk is that complex traits and ecosystem interactions are hard to capture in models. Plants exist in the real world with microbes, pollinators, unexpected weather events. An AI might predict a variety will excel, but reality could prove otherwise if there’s an overlooked variable. There’s also the chance of over-optimization: a plant perfectly bred for one set of conditions might falter if conditions change slightly. Breeders will need to make sure they aren’t just breeding formula-one race car plants that perform amazingly in a controlled setting but are fragile outside those parameters.
- Ethical and social considerations: Who owns the algorithms that design our food, and who benefits? These AI breeding technologies will likely be patented and owned by private firms. That could concentrate even more power in the hands of a few large agri-tech companies. If a handful of algorithms end up designing a significant portion of the world’s crops, it raises questions about food sovereignty. Farmers, especially those in developing countries, might become dependent on seeds resulting from processes they have no say in. On the other hand, if done collaboratively, AI breeding could be democratized – imagine open-source algorithms that national breeding programs or small startups could use to develop crops for local needs. One positive sign is that players like Heritable and Inari are actively partnering with others rather than keeping tech siloed; Inari’s CFO even said problems like food security and climate are “far too big for any one or two organizations to address alone. Broad collaboration is a necessity.”. Ethically, there’s also the matter of transparency: an AI might create a new variety, but can it explain why that plant is the way it is? Breeders usually have to register new varieties and describe their lineage and traits. If AI selections become too opaque, regulators and consumers might demand more insight into the process (this touches on the broader AI “black box” issue). And while consumers probably won’t object to “AI-bred” fruits the way some do to “GMO” labels – since there’s no foreign DNA involved – clear communication will be important to avoid misunderstanding.
- Economic impacts: The introduction of AI-bred crops could disrupt agricultural markets. For farmers, having higher-yielding or easier-to-grow varieties is generally a boon – it can improve profitability. But consider a scenario: if indoor-grown strawberries become extremely efficient and popular, what happens to the traditional strawberry farms in, say, California or Florida? There could be shifts in regional production. Some traditional growers might suffer if market prices drop or if consumer preferences shift to berries grown closer to home. However, it might also create new opportunities – those same farmers could adopt improved varieties or even invest in protected culture themselves. The companies that move fastest in adopting AI-bred seeds might gain competitive advantages. We could see new leaders in the seed industry emerging if tech-forward startups out-innovate the old guard. At the same time, major seed corporations have deep pockets and are incorporating AI too, so it might become a race fueled by acquisitions and partnerships. The fact that Heritable, a young startup, is collaborating with an established breeder and a produce company suggests a cooperative path, but one can imagine if their approach succeeds, larger firms will either partner, imitate, or attempt to buy them out. Another economic angle is cost: developing a new variety through conventional means costs millions of dollars over many years. If AI can trim those costs and timelines, it could lower the barriers to entry for creating specialized crops (for niche markets, local climates, etc.), potentially decentralizing some innovation. However, the flipside is the software and data needed for AI breeding might be expensive – requiring high-performance computing and expertise – which not everyone can afford. There’s a risk of a digital divide in agriculture, where those with access to big data and AI gain even more advantage over those without.
- Technological challenges and risks: Despite the excitement, it’s worth remembering that plants are not as easily predictable as, say, chess moves or stock prices. Biological systems have inherent randomness and emergent properties. An AI model is only as good as the data it’s trained on. If there are biases or gaps in the data (for instance, maybe all historical breeding data comes from moderate climates, and the model has never seen what extreme heat does), the predictions could fail under new scenarios – exactly when we need innovation the most (like unprecedented climate conditions). Ensuring that models are trained on diverse, representative datasets is crucial. There’s also the risk of over-reliance on virtual predictions. Breeders might be tempted to trust the computer so much that they conduct fewer real-world trials. That could be dangerous; field testing and validation must remain a cornerstone. The ideal is a tight feedback loop: use AI to pick better candidates, but then rigorously test those candidates and feed the results back into the model to improve it continuously. Another risk: intellectual property battles. If an AI recombines existing genes in a novel way, who owns the result? Patent law in plant breeding can be thorny. There may be disputes over whether the innovation lies in the algorithm or in the plant’s DNA or both. On the tech side itself, one challenge is making AI models explainable and trusted by breeders. If a model suggests crossing two very genetically distant lines because it predicts a great outcome, a breeder might be skeptical – it helps if the AI can highlight which genes or traits are the drivers of that prediction. Developing user-friendly tools will determine how broadly adopted these techniques become. The good news is that research communities are already working on this; for example, efforts are underway to integrate environmental data and machine learning to improve genomic selection in crops, and to create interfaces that let breeders use AI without needing a PhD in data science.
As we stand at this crossroads of agriculture and artificial intelligence, the stakes are high but so is the potential payoff. The AI-driven strawberry breeding program by Heritable, CIV, and Mastronardi is in many ways a microcosm of the larger trend – a testbed that will be watched closely. If, two or three years from now, supermarkets start carrying a delicious new strawberry variety that was developed in half the usual time and thrives in local greenhouses, it will validate not just one partnership but an entire approach to innovation. And if it falls short, that too will impart valuable lessons on the limits of technology in agriculture’s unpredictable theatre.
A New Green Revolution in the Making?
It’s tempting to draw parallels to history. In the mid-20th century, the Green Revolution transformed global agriculture through improved plant breeds (among other inputs like fertilizers). Those advances were largely driven by empirical science and painstaking field trials by the likes of Norman Borlaug in wheat and Henry Beachell in rice. Today, we may be on the cusp of a new green revolution – one driven by algorithms, big data, and cross-industry collaboration. “Our work with Heritable Agriculture and CIV not only reimagines how we breed strawberries but also sets the stage for a new era of greenhouse crop innovation,” said Paul Mastronardi. The stage is indeed set for a new era, and not just in greenhouses.
The challenges facing our food systems are urgent: the need to grow more with less, to adapt to climate upheavals, to nourish a growing population sustainably. No single solution will achieve all that, but the fusion of artificial intelligence with the biological intelligence of seeds and farmers may be a significant part of the answer. By harnessing AI to augment human expertise – not replace it, but amplify it – we can explore possibilities in crop improvement at a scale and speed previously unimaginable. As one plant breeding expert, Jianming Yu of Iowa State University, put it, “AI provides a new lens to bridge science and practice” in crop improvement, though he cautions that at large scales, we are just at the beginning of figuring out how best to use these tools. The coming years will likely bring a flurry of experimentation, successes, and yes, some failures, as the industry learns how to marry Silicon Valley algorithms with the dirt-under-nails reality of farming.
In a way, the strawberry – a small, delicate fruit – is a fitting symbol. It has always required careful nurturing and clever horticulture to bring out its best, from the monastery gardeners of the 14th century who first domesticated wild strawberries, to the modern growers coaxing berries in winter greenhouses. Now, that same care and cleverness is being encoded in computer models. If those models succeed, a strawberry picked in a January greenhouse could embody a host of innovations: the genomics of an Italian seed bank, the machine learning of a California tech lab, the horticultural savvy of a Canadian grower, all coming together to create something new and delightful. And if that can happen for a strawberry, it can happen for many other crops we depend on.
For now, we await the first fruits – quite literally – of this AI-driven breeding effort. As the seedlings from Heritable and CIV’s collaborative crosses grow in test greenhouses over the next year, scientists will be watching whether reality matches the AI’s predictions. The excitement in agtech circles is palpable. There’s talk that this could be one of those inflection points in agriculture, where future historians will say that the mid-2020s were when breeders started routinely collaborating with computers and data scientists, not just agronomists and geneticists. Skeptics, of course, will urge caution: nature has a way of humbling our high-tech ambitions. But even the skeptics are eager to see what emerges.
In the end, if the flavor of a strawberry can be improved by an algorithm – and delivered to a consumer with all the juiciness intact – it bodes well for what else we might achieve. AI won’t replace the sun, soil, and skill that good farming requires, but it might help us use them more intelligently. The promise isn’t just a better berry or a single company’s success; it’s a blueprint for how we might intelligently design a more resilient and abundant food future. That’s an idea worth savoring, perhaps as much as the berries themselves.